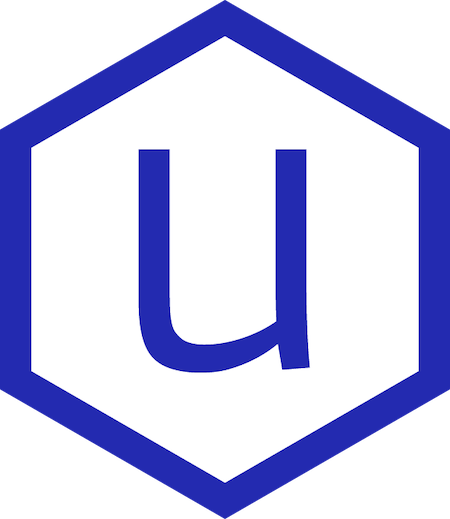
Ensuring data integrity in enterprise R&D laboratories is a multifaceted challenge, compounded by the rapid evolution of technology and increasing external pressures. Implementing robust data management strategies and advanced tools and technologies is essential for R&D teams to build trust in their data and processes. Strengthening data integrity safeguards research against scrutiny and empowers teams to focus more on innovation and lab work, accelerating scientific discovery within large organizations.
Key Factors Influencing Data Integrity in Enterprise R&D Laboratories
While numerous factors influence data integrity in enterprise R&D environments, three are particularly critical: organizational culture, technology, and continuous training.
I. Culture: Fostering Accountability and Quality in Enterprise R&D
Creating a culture of accountability, quality, and security is vital for maintaining data integrity across large R&D organizations. However, researchers often face significant pressure to deliver results, whether driven by the need to publish findings or meet aggressive project deadlines. This urgency can sometimes lead to shortcuts that compromise data integrity, potentially causing costly setbacks such as the need to repeat experiments, reprocess data, or even retract findings. Moreover, such lapses can undermine product safety and efficacy, jeopardize patents and funding, and damage the organization’s reputation.
To mitigate these risks, enterprise R&D organizations must balance their milestone-driven objectives with a steadfast commitment to data integrity. This requires holding researchers accountable for conducting reproducible experiments that can withstand rigorous scrutiny. However, accountability must be paired with the provision of the right tools and technologies that enable researchers to adhere to best practices in data management. Ultimately, it is the organization's responsibility to invest in the necessary infrastructure that empowers its researchers to maintain high standards of data integrity while achieving their scientific goals.
II. Technology: Implementing Comprehensive Data Management in R&D Laboratories
a. Data Integrity and Structured Data: Distinguishing and Connecting the Concepts
In enterprise R&D, it’s crucial to distinguish between data integrity and structured data while understanding how they are interconnected to support effective research and innovation.
Data Integrity refers to data's accuracy, consistency, and reliability throughout its lifecycle. It ensures the data remains unchanged from its source and accurately reflects the intended information. Maintaining data integrity involves implementing rigorous validation processes, error-checking mechanisms, and adherence to data governance standards to prevent corruption or unauthorized alterations.
Structured Data, however, refers to data organized in a specific format or schema, typically in rows and columns, making it easily searchable and analyzable. Structured data is often stored in databases, spreadsheets, or tables, where each entry follows a consistent format. This organization makes it straightforward to manage and analyze, especially when leveraging advanced tools like AI and machine learning.
While structured data provides a framework that simplifies data management, having structured data alone does not ensure data integrity. Structured data can still suffer integrity issues if incomplete, corrupted, or inaccurately entered. Therefore, though distinct, structured data and data integrity must work hand in hand to maximize the quality and utility of the data used in R&D processes.
b. The Role of Structured Data in Supporting Data Integrity
The organization inherent in structured data aids in maintaining data integrity by making it easier to track, validate, and manage data throughout its lifecycle. For instance, structured data allows for the implementation of checks and balances that ensure the accuracy and consistency of the data as it moves through various stages of collection, storage, analysis, and reporting.
A critical component of this is creating a complete digital data-provenance trail that documents every step of the data's journey, including layers of processing and analysis by different users.
This traceability is vital for facilitating audits, recovering data, and ensuring reproducibility. As funding and regulatory bodies, such as the NIH and FDA, increasingly emphasize FAIR (Findable, Accessible, Interoperable, and Reusable) data principles and research reproducibility, it becomes even more imperative for large R&D organizations to adopt flexible and scalable technology that enables them to adhere to best practices in documenting their processes and managing their data.
c. Structured Data and AI: The Foundation for Accurate and Effective AI Implementations
Structured data plays a pivotal role in successfully implementing AI within enterprise R&D environments. AI systems depend heavily on structured data to recognize patterns, make predictions, and generate insights. For example, AI models can predict new materials' properties and potential applications in materials discovery based on historically structured data. However, if the data lacks integrity—meaning it is incomplete, inconsistent, or inaccurate—the predictions made by AI could lead to pursuing ineffective materials, ultimately wasting time and resources.
Moreover, the transparency and traceability of AI-driven decisions depend heavily on data integrity. Researchers and regulatory bodies need to be able to trace AI-generated outcomes back to the original data and processes to validate findings. This traceability is crucial, especially as AI plays an increasing role in decision-making within regulated environments like chemicals or material science. Structured data ensures that AI models can be audited, their decisions explained, and, if necessary, corrected, maintaining trust in the AI systems deployed across R&D functions.
To fully leverage AI's potential in enterprise R&D, organizations must ensure that their data integrity practices are robust. This includes maintaining clean, consistent and well-documented structured data sets free from errors or biases. Effective data governance policies and advanced data management tools ensure that AI models are trained on high-quality data and operate within a framework that allows for continuous improvement and refinement.
By focusing on structured data and data integrity, R&D organizations can maximize the effectiveness of AI, leading to more accurate predictions, faster innovation cycles, and, ultimately, a competitive edge in their respective industries. Data integrity becomes the bedrock upon which successful AI implementation is built, enabling organizations to harness the full power of AI to drive forward their research and development initiatives.
III. Training: Building a Culture of Continuous Improvement in Enterprise R&D
Data integrity is a shared responsibility that requires ongoing education and training to cultivate a quality culture within enterprise R&D laboratories. Every research team member, from junior scientists to senior executives, must understand their role in maintaining research integrity and security. This begins with setting clear expectations at all levels and providing targeted training on tools, processes, and responsibilities.
For researchers, training should encompass the technical aspects of data management and the importance of meticulous experimental planning, accurate data recording, and thorough documentation. Good record-keeping is crucial for ensuring reproducibility and credibility and plays a key role in onboarding new team members and managing transitions within the lab. At the management level, lab leaders should establish clear data management requirements at the outset of every project and create channels for reporting issues as they arise.
An enterprise R&D organization’s commitment to data integrity—complemented by the use of structured data—should extend beyond technology adoption to include a focus on the human element. Both are essential for building a trustworthy research process that delivers reliable, reproducible results, ultimately driving innovation.
Enhancing Data Integrity in Your Enterprise R&D Operations
At Uncountable, we understand the complexities of ensuring data integrity—supported by structured data—within enterprise R&D laboratories. Our team of experts is dedicated to helping organizations overcome these challenges by providing cutting-edge tools and strategies tailored to the unique needs of R&D professionals. By partnering with Uncountable, you can strengthen your data management practices, enhance research reproducibility, and ensure that your AI-driven initiatives are as accurate and effective as possible, driving innovation within your organization.